We Expect Humans To Be Accountable. We Should Expect No Less Of AI
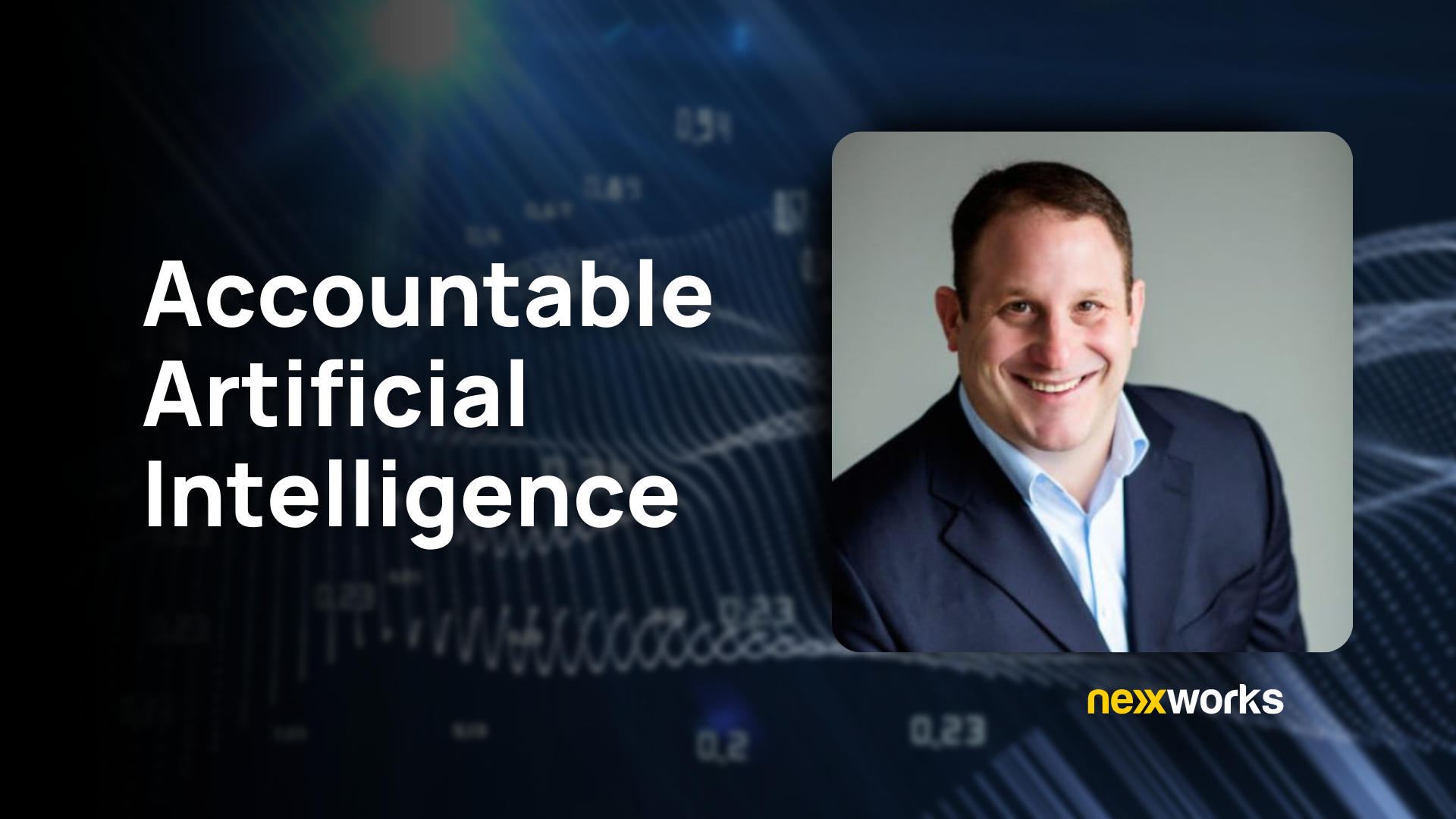
About ten years ago, IBM invited me to talk with some key members on the Watson team, when the triumph of creating a machine that could beat the best human players at the game show Jeopardy! was still fresh. I wrote in Forbes at the time that we were entering a new era of cognitive collaboration between humans, computers and other humans.
One thing that struck me was how similar the moment seemed to how aviation legend Chuck Yeager described the advent of flying-by-wire, four decades earlier, in which pilots no longer would operate aircraft, but interface with a computer that flew the plane. Many of the macho “flyboys” weren’t able to trust the machines and couldn’t adapt.
Now, with the launch of ChatGPT, Bill Gates has announced that the age of AI has begun and, much like those old flyboys, we’re all going to struggle to adapt. Our success will not only rely on our ability to learn new skills and work in new ways, but the extent to which we are able to trust our machine collaborators. To reach its potential, AI will need to become accountable.
Recognizing Data Bias
With humans, we work diligently to construct safe and constructive learning environments. We design curriculums, carefully selecting materials, instructors and students to try and get the right mix of information and social dynamics. We go to all this trouble because we understand that the environment we create greatly influences the learning experience.
Machines also have a learning environment called a “corpus.” If, for example, you want to teach an algorithm to recognize cats, you expose it to thousands of pictures of cats. In time, it figures out how to tell the difference between, say, a cat and a dog. Much like with human beings, it is through learning from these experiences that algorithms become useful.
However, the process can go horribly awry. A famous case is Microsoft’s Tay, a Twitter bot that the company unleashed on the microblogging platform in 2016. In under a day, Tay went from being friendly and casual (“humans are super cool”) to downright scary, (“Hitler was right and I hate Jews”). It was profoundly disturbing.
Bias in the learning corpus is far more common than we often realize. Do an image search for the word “professional haircut” and you will get almost exclusively pictures of white men. Do the same for “unprofessional haircut” and you will see much more racial and gender diversity.
It’s not hard to figure out why this happens. Editors writing articles about haircuts portray white men in one way and other genders and races in another. When we query machines, we inevitably find our own biases baked in.
Accounting For Algorithmic Bias
A second major source of bias results from how decision-making models are designed. Consider the case of Sarah Wysocki, a fifth grade teacher who — despite being lauded by parents, students, and administrators alike — was fired from the D.C. school district because an algorithm judged her performance to be sub-par. Why? It’s not exactly clear, because the system was too complex to be understood by those who fired her.
Yet it’s not hard to imagine how it could happen. If a teacher’s ability is evaluated based on test scores, then other aspects of performance, such as taking on children with learning differences or emotional problems, would fail to register, or even unfairly penalize them. Good human managers recognize outliers, algorithms generally aren’t designed that way.
In other cases, models are constructed according to what data is easiest to acquire or the model is overfit to a specific set of cases and is then applied too broadly. In 2013, Google Flu Trends predicted almost double as many cases there actually were. What appears to have happened is that increased media coverage about Google Flu Trends led to more searches by people who weren’t sick. The algorithm was never designed to take itself into account.
The simple fact is that an algorithm must be designed in one way or another. Every possible contingency cannot be pursued. Choices have to be made and bias will inevitably creep in. Mistakes happen. The key is not to eliminate error, but to make our systems accountable through, explainability, auditability and transparency.
To Build An Era Of Cognitive Collaboration We First Need To Build Trust
In 2020, Ofqual, the authority that administers A-Level college entrance exams in the UK, found itself mired in scandal. Unable to hold live exams because of Covid-19, it designed and employed an algorithm that based scores partly on the historical performance of the schools students attended with the unintended consequence that already disadvantaged students found themselves further penalized by artificially deflated scores.
The outcry was immediate, but in a sense the Ofqual case is a happy story. Because the agency was transparent about how the algorithm was constructed, the source of the bias was quickly revealed, corrective action was taken in a timely manner, and much of the damage was likely mitigated. As Linus’s Law advises, “given enough eyeballs, all bugs are shallow.”
The age of artificial intelligence requires us to collaborate with machines, leveraging their capabilities to better serve other humans. To make that collaboration successful, however, it needs to take place in an atmosphere of trust. Machines, just like humans, need to be held accountable, their decisions and insights can’t be a “black box.” We need to be able to understand where their judgments come from and how they’re decisions are being made.
Senator Schumer is reportedly working on legislation that will promote more transparency, but that is only a start. The real change has to come from within ourselves and how we see our relationships with the machines we create. Marshall McLuhan wrote that media are extensions of man and the same can be said for technology. Our machines inherit our human weaknesses and frailties. We need to make allowances for that.
This article was first published on Greg's personal website.
Read that and more here.

%20(1).png)